Boost Your Data Understanding: What Is Pointwise Mutual Information?
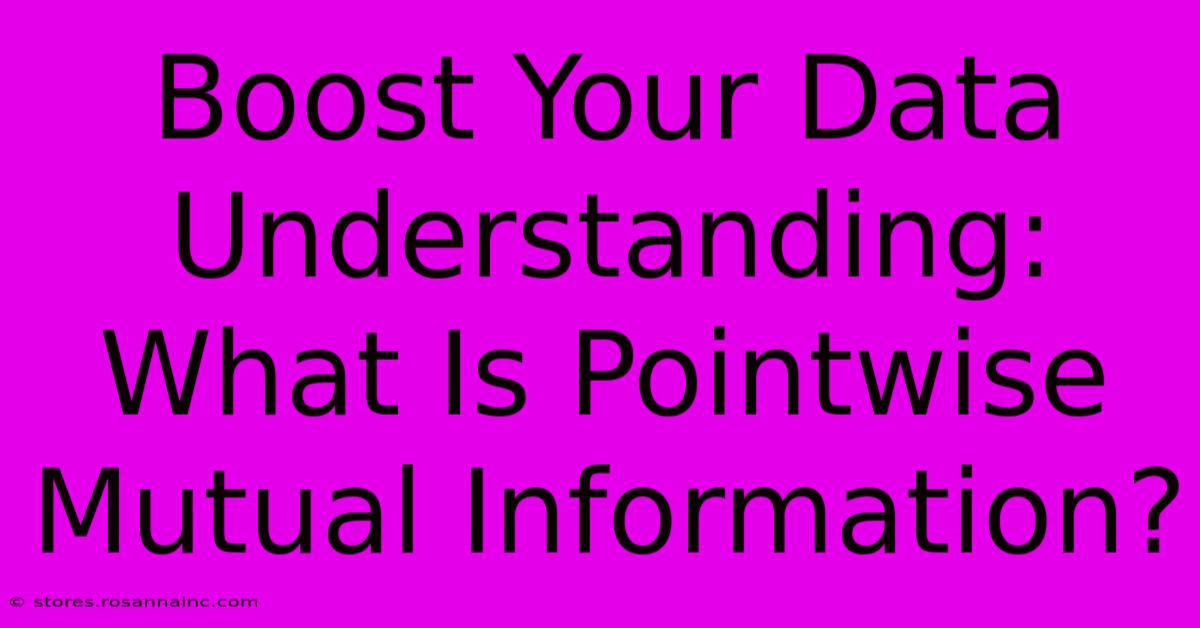
Table of Contents
Boost Your Data Understanding: What is Pointwise Mutual Information?
Understanding your data is crucial for any successful data science project. One powerful tool that can significantly enhance your comprehension is Pointwise Mutual Information (PMI). This metric helps quantify the relationship between two events, revealing how much knowing about one event changes the likelihood of the other. This article will demystify PMI, explaining its calculation, applications, and limitations.
What is Pointwise Mutual Information (PMI)?
In simpler terms, PMI measures the statistical association between two discrete random variables. A high PMI suggests a strong relationship; a low or negative PMI indicates a weak or inverse relationship. It's essentially a measure of how much more likely one event is given that another event has occurred.
Imagine you're analyzing customer purchase data. You might want to know if the purchase of "laptop" is related to the purchase of "laptop bag." PMI can help you quantify this relationship. If customers who buy laptops are significantly more likely to also buy laptop bags than the general population, the PMI between these two items will be high.
Mathematically, PMI is defined as:
PMI(x, y) = log₂[P(x, y) / (P(x)P(y))]
Where:
- P(x, y) is the joint probability of events x and y occurring together.
- P(x) is the probability of event x occurring.
- P(y) is the probability of event y occurring.
- log₂ is the base-2 logarithm, resulting in a PMI value expressed in bits.
Interpreting PMI Values
- PMI > 0: Indicates a positive association between x and y. The occurrence of one event increases the likelihood of the other.
- PMI = 0: Indicates no association between x and y. The events are independent.
- PMI < 0: Indicates a negative association (or inverse relationship) between x and y. The occurrence of one event decreases the likelihood of the other.
Applications of Pointwise Mutual Information
PMI finds applications in various fields, including:
- Natural Language Processing (NLP): Identifying collocations (words that frequently appear together), improving word embeddings, and building language models.
- Information Retrieval: Determining the relevance of documents to search queries.
- Bioinformatics: Analyzing gene expression data and identifying relationships between genes.
- Recommendation Systems: Suggesting items that are frequently purchased together.
Example in NLP:
Consider the words "artificial" and "intelligence". If their PMI is high, it suggests a strong association, helping to identify this phrase as a common collocation. This is valuable for tasks like text summarization or keyword extraction.
Limitations of Pointwise Mutual Information
While PMI is a powerful tool, it's essential to be aware of its limitations:
- Sensitivity to Frequency: PMI can be biased towards frequent events. Rare events might have artificially inflated PMI scores simply due to low probabilities.
- Data Sparsity: With limited data, probabilities might be inaccurate, leading to unreliable PMI estimations. Smoothing techniques can help mitigate this.
- Doesn't capture complex relationships: PMI is limited to pairwise relationships. It doesn't capture higher-order interactions between multiple events.
Boosting Your Data Analysis with PMI
Pointwise Mutual Information provides a valuable method for uncovering relationships within your data. By understanding its calculation, interpretation, and limitations, you can leverage its power to gain deeper insights and improve your data analysis workflows. Remember to consider the context of your data and use PMI in conjunction with other analysis techniques for a comprehensive understanding. Addressing the limitations by using appropriate data preprocessing and smoothing techniques will further enhance the reliability of your results. Ultimately, mastering PMI will equip you with a crucial tool in your data scientist's arsenal.
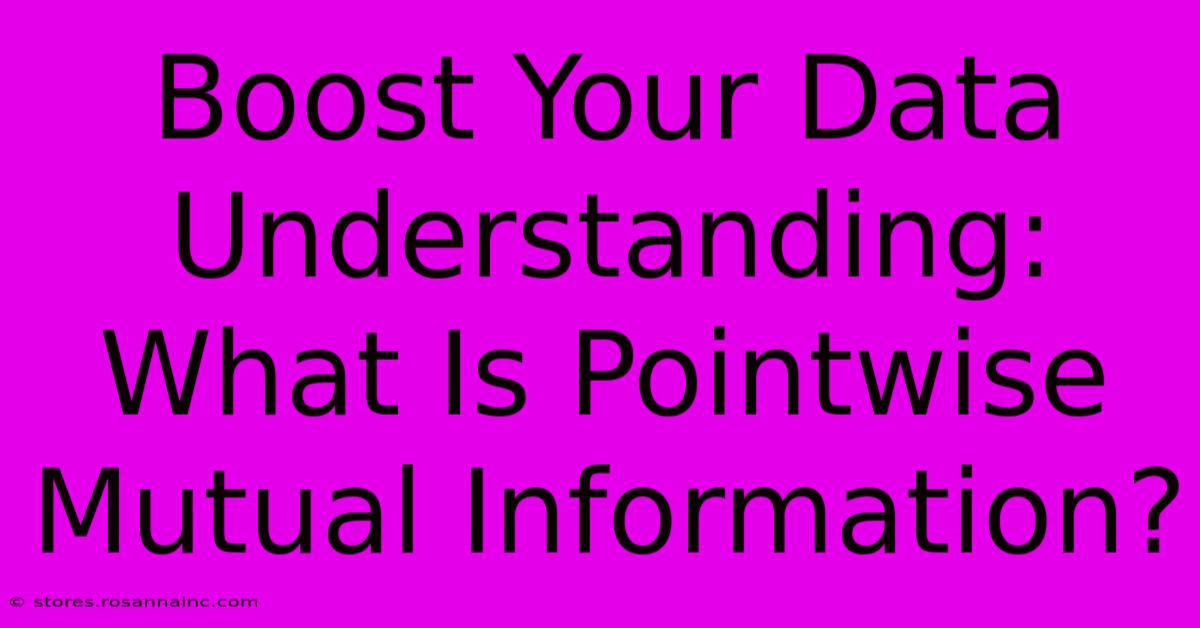
Thank you for visiting our website wich cover about Boost Your Data Understanding: What Is Pointwise Mutual Information?. We hope the information provided has been useful to you. Feel free to contact us if you have any questions or need further assistance. See you next time and dont miss to bookmark.
Featured Posts
-
Axl Rose Owns Guns N Roses What It Means For Fans
Feb 10, 2025
-
Leave The Ordinary Behind Bury Me At Makeout Creek
Feb 10, 2025
-
Super Bowl 2024 Time Countdown Is On
Feb 10, 2025
-
Interview With A Vampire Book Everything You Need To Know Before You Read
Feb 10, 2025
-
Rancho Santa Fe Ca Where Luxury Meets Laid Back Living
Feb 10, 2025