Conquering Complex Data With The Generalized Method Of Moments
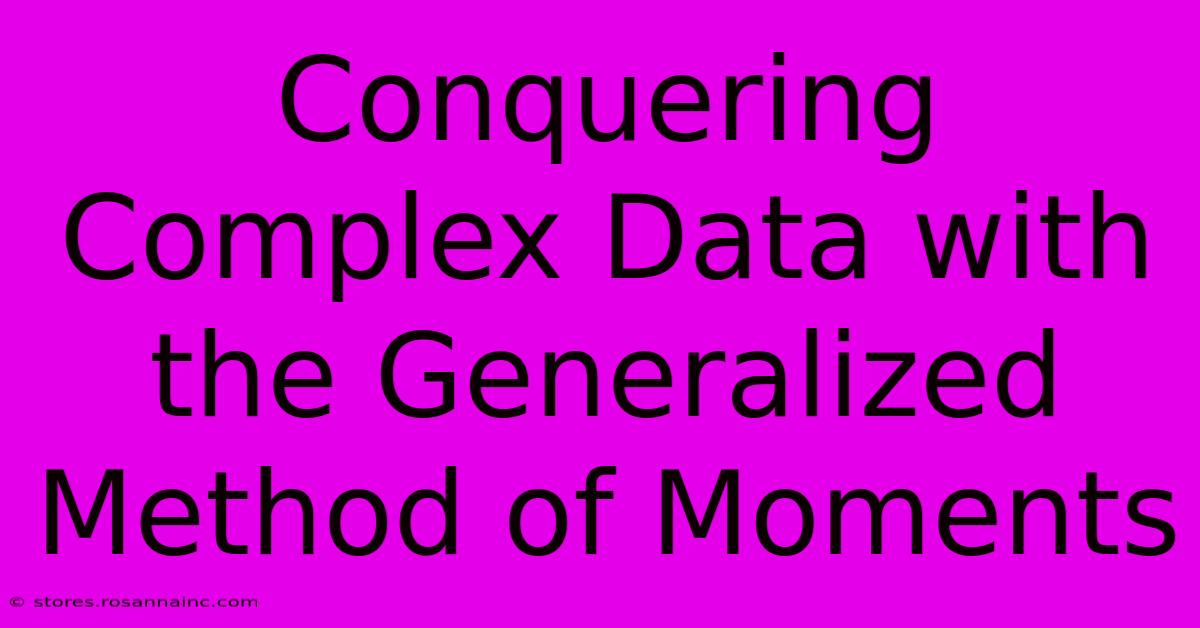
Table of Contents
Conquering Complex Data with the Generalized Method of Moments (GMM)
The world is awash in data. But raw data, without the right analytical tools, is just noise. When facing complex datasets riddled with challenges like endogeneity, missing data, or non-linear relationships, traditional econometric methods often fall short. This is where the Generalized Method of Moments (GMM) emerges as a powerful and versatile solution. This article explores the applications, advantages, and nuances of GMM, empowering you to unlock the insights hidden within even the most intricate datasets.
Understanding the Power of GMM
GMM is a powerful statistical technique used to estimate parameters in econometric models. Unlike traditional methods that rely on strong distributional assumptions, GMM leverages a set of moment conditions. These conditions represent relationships between the model's parameters and the data's moments (e.g., means, variances, covariances). By exploiting these relationships, GMM provides consistent and asymptotically normal estimators even when faced with complex data structures.
Key Advantages of GMM:
- Robustness: GMM handles data violations of strict assumptions, such as heteroskedasticity and autocorrelation, which often plague traditional methods like Ordinary Least Squares (OLS).
- Flexibility: It adapts to a wide variety of models, including dynamic panel data models, non-linear models, and models with endogenous variables.
- Efficiency: Under correct specification, GMM estimators are asymptotically efficient. This means that they extract the maximum amount of information from the data to obtain the most precise estimates.
- Wide Applicability: GMM finds applications in various fields, including economics, finance, and engineering.
Tackling Endogeneity with GMM
One of the most significant advantages of GMM is its ability to address endogeneity, a common problem where explanatory variables are correlated with the error term. This correlation leads to biased and inconsistent OLS estimates. GMM skillfully navigates this issue by using instrumental variables (IVs). These IVs are variables correlated with the endogenous regressors but uncorrelated with the error term. By incorporating these IVs into the moment conditions, GMM generates consistent estimates even in the presence of endogeneity.
Implementing GMM: A Step-by-Step Approach
While the underlying mathematics can be complex, the implementation of GMM is often straightforward using statistical software packages like Stata, R, or Python. The general steps involved are:
- Specify the model: Define the econometric model and its parameters.
- Determine moment conditions: Identify relevant moment conditions based on the model's theoretical properties and available data. This step requires careful consideration of the model's assumptions and potential sources of bias.
- Choose instrumental variables (if necessary): Select appropriate instrumental variables if dealing with endogenous variables. The choice of IVs is crucial for the validity and efficiency of the GMM estimates.
- Estimate the parameters: Use a GMM estimation procedure to obtain the model's parameter estimates. The procedure involves minimizing a GMM objective function, often based on a weighted average of the sample moments.
- Test for over-identification: Perform a test to check whether the number of moment conditions exceeds the number of parameters. This is essential for evaluating the model's overall validity.
Beyond the Basics: Advanced GMM Techniques
The basic GMM framework can be extended to handle various complexities. These include:
- Two-Step GMM: This approach involves estimating the optimal weighting matrix in a first step and then using this matrix in a second step to obtain more efficient estimates.
- Continuous Updating GMM (CUE): CUE provides a more efficient estimation method by iteratively updating the weighting matrix.
- Generalized Empirical Likelihood (GEL): GEL offers an alternative approach to GMM that can be more robust to misspecification.
Conclusion: Mastering the Power of GMM
The Generalized Method of Moments provides a powerful and flexible toolkit for navigating the complexities of modern datasets. By intelligently employing moment conditions and instrumental variables, researchers and analysts can overcome the limitations of traditional methods and extract valuable insights from even the most challenging data. While the implementation requires a solid understanding of econometric principles, the rewards – robust, efficient, and reliable estimates – are well worth the effort. Mastering GMM empowers you to conquer complex data and unlock the hidden knowledge within.
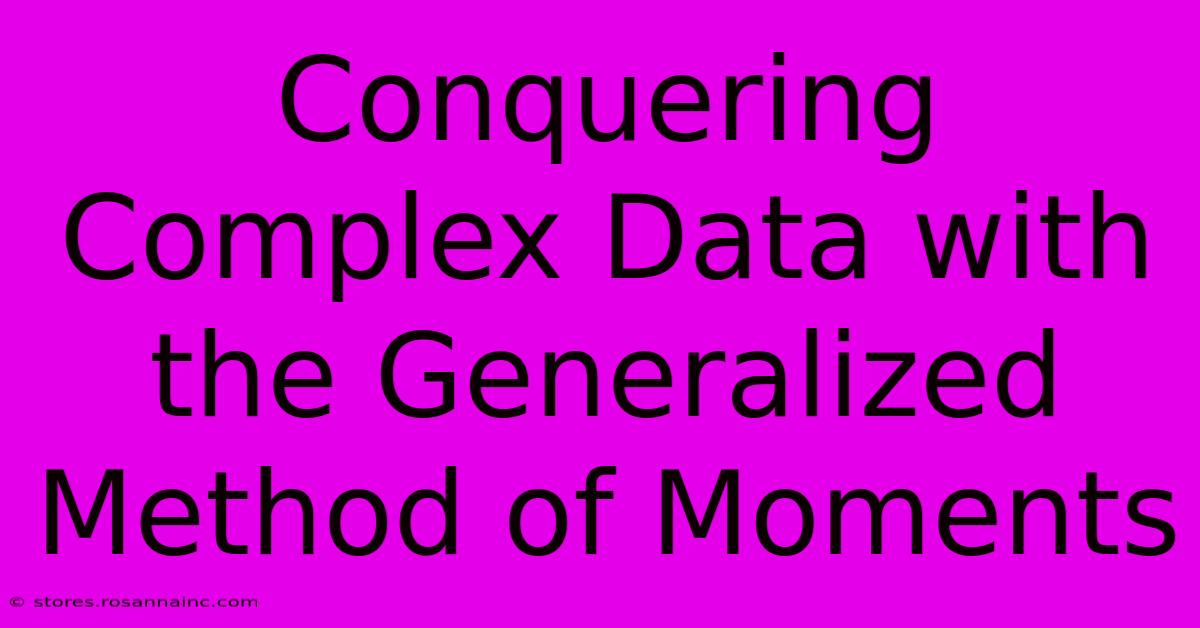
Thank you for visiting our website wich cover about Conquering Complex Data With The Generalized Method Of Moments. We hope the information provided has been useful to you. Feel free to contact us if you have any questions or need further assistance. See you next time and dont miss to bookmark.
Featured Posts
-
Lily Rose Depp From Chanel To Screen Her Evolution As An Artist
Feb 09, 2025
-
Demande Kanye West Post Scandale Censori
Feb 09, 2025
-
Middlesex College Nj Is Your Tuition Money Going To The Right Place
Feb 09, 2025
-
Your It Ends With Us Showtimes Guide Hassle Free Booking
Feb 09, 2025
-
Forget The Records Kelces Nfl History Changes Everything
Feb 09, 2025