GMM: A Smarter Way To Estimate
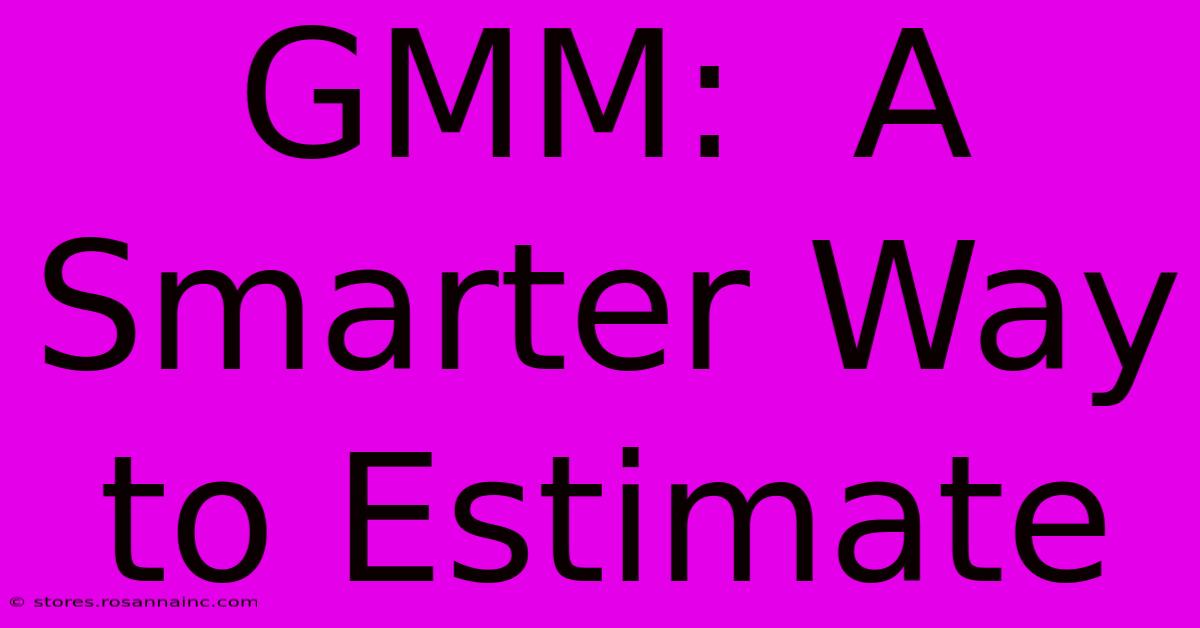
Table of Contents
GMM: A Smarter Way to Estimate
Generalized Method of Moments (GMM) is a powerful statistical technique used to estimate parameters in econometric and statistical models. Unlike traditional methods like Ordinary Least Squares (OLS), GMM is particularly useful when dealing with models that have more equations than parameters (overidentified) or when assumptions underlying OLS, such as strict exogeneity, are violated. This article will delve into the core principles of GMM, highlighting its advantages and providing practical insights.
Understanding the Essence of GMM
GMM's strength lies in its flexibility. It leverages moment conditions, which are theoretical relationships between the model's parameters and the observable data. These conditions are expressed as expectations, meaning that certain functions of the data should have an expected value of zero if the model is correctly specified.
For example, consider a simple linear model where the error term is not necessarily uncorrelated with the independent variables. OLS would yield biased and inconsistent estimates in this scenario. GMM, however, can still provide consistent estimates by cleverly using instrumental variables to satisfy the moment conditions, even in the presence of endogeneity.
Key Advantages of GMM:
- Handles Endogeneity: GMM excels in situations where explanatory variables are correlated with the error term (endogeneity). This is a common problem in many economic and social science applications.
- Flexibility in Model Specification: It can accommodate a wide range of model specifications, including nonlinear models and models with various forms of heteroskedasticity.
- Overidentified Models: GMM can effectively handle overidentified models, where the number of moment conditions exceeds the number of parameters to be estimated. This allows for a more robust estimation and provides a test of the model's specification.
- Consistent Estimation: Under relatively weak assumptions, GMM provides consistent parameter estimates.
The Mechanics of GMM Estimation
GMM works by minimizing a distance metric between the sample moments and their theoretical counterparts (zero). This minimization process involves a weighting matrix, which plays a crucial role in the efficiency of the estimates. The optimal weighting matrix is based on the asymptotic variance of the sample moments.
The estimation process typically involves these steps:
- Specify the Moment Conditions: Define the theoretical relationships between the parameters and the data, expressing them as moment conditions with an expected value of zero.
- Choose Instrumental Variables (if necessary): If endogeneity is a concern, select instrumental variables that are correlated with the endogenous variables but uncorrelated with the error term.
- Estimate the Sample Moments: Calculate the sample averages of the moment conditions.
- Select a Weighting Matrix: The choice of weighting matrix significantly affects the efficiency of the estimator. The optimal weighting matrix is a consistent estimate of the inverse of the variance-covariance matrix of the sample moments.
- Minimize the Distance: Minimize the weighted distance between the sample moments and their theoretical values (zero). This minimization is usually done using iterative numerical methods.
- Obtain Parameter Estimates and Standard Errors: The solution to the minimization yields the GMM parameter estimates. Standard errors are then computed to assess the precision of the estimates.
J-Test: Assessing Model Specification
A crucial aspect of GMM is the J-test, also known as the Hansen test. This test assesses the overall validity of the model's specification by examining whether the moment conditions are satisfied. A statistically significant J-statistic indicates that the model is misspecified, suggesting that some of the moment conditions are not met.
GMM in Practice
GMM has found wide application across numerous fields:
- Economics: Estimating dynamic panel data models, analyzing simultaneous equations, and addressing endogeneity issues in various economic contexts.
- Finance: Modeling asset pricing, estimating risk premia, and analyzing portfolio performance.
- Biostatistics and Epidemiology: Estimating parameters in complex statistical models, particularly where the assumptions of standard methods are not met.
Conclusion
GMM offers a powerful and flexible approach to parameter estimation in a wide range of statistical and econometric models. Its ability to handle endogeneity, its suitability for overidentified models, and its robustness make it an indispensable tool for researchers and analysts across many disciplines. While the implementation can be computationally intensive, the benefits in terms of consistent and efficient estimation often outweigh the costs. Understanding the core principles and limitations of GMM is crucial for proper application and interpretation of the results.
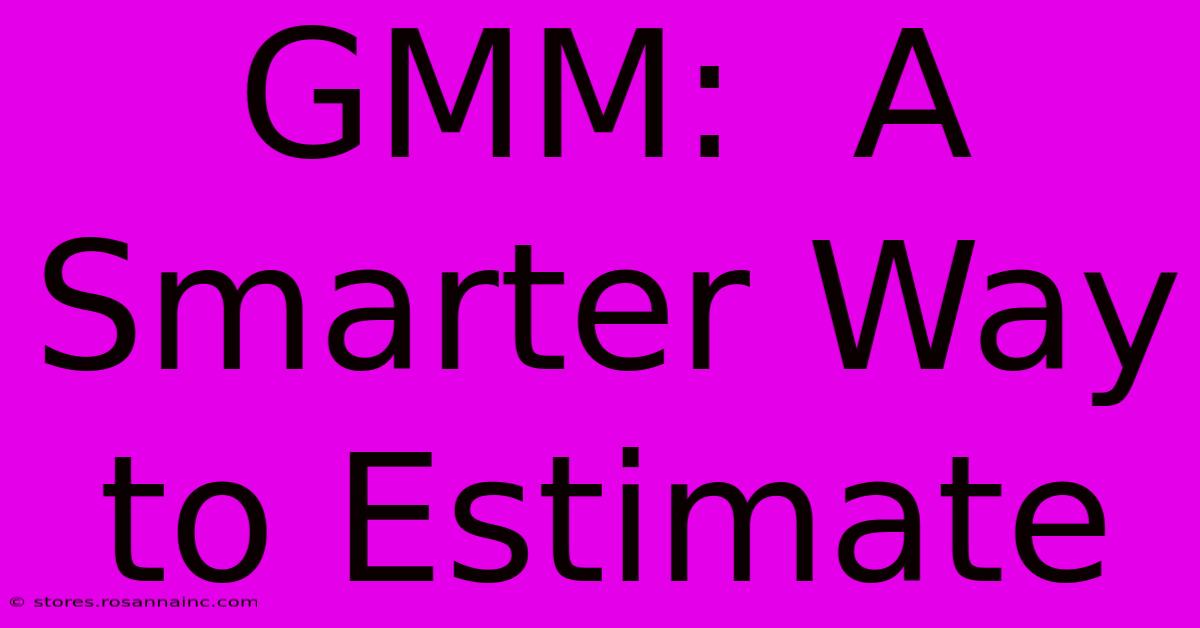
Thank you for visiting our website wich cover about GMM: A Smarter Way To Estimate. We hope the information provided has been useful to you. Feel free to contact us if you have any questions or need further assistance. See you next time and dont miss to bookmark.
Featured Posts
-
Firth Of Forth Estuary A Timeless Treasure
Feb 11, 2025
-
Taylor Swift Producer Drops Major Hint New Album 2024 Confirmed
Feb 11, 2025
-
How Much Is Rick Moranis Worth After Leaving Hollywood
Feb 11, 2025
-
Ligue Des Champions Phase De Groupes
Feb 11, 2025
-
Overcome Prejudice The Inspiring Message Of El Jorobado De Notre Dame
Feb 11, 2025