Pointwise Mutual Information: The Secret To Deeper Data Analysis
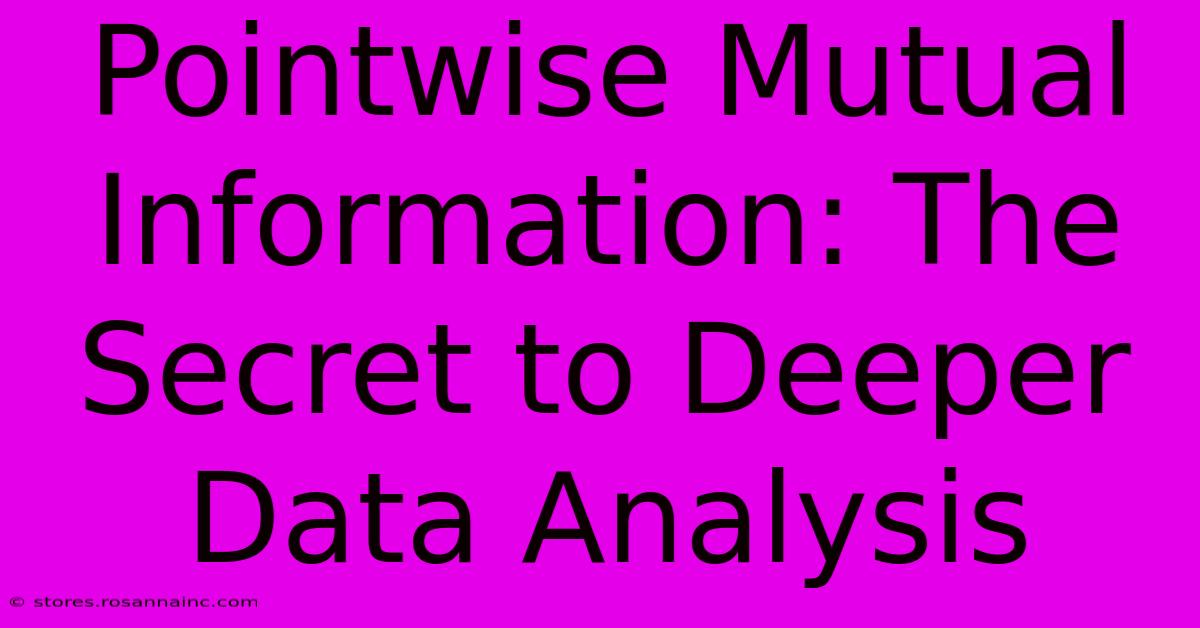
Table of Contents
Pointwise Mutual Information: The Secret to Deeper Data Analysis
Unlocking hidden relationships within your data is crucial for making informed decisions. While correlation analysis offers a valuable starting point, it often falls short in revealing the true nuances of complex datasets. This is where Pointwise Mutual Information (PMI) steps in, providing a powerful tool for uncovering subtle dependencies and enriching your data analysis. This article delves into the intricacies of PMI, explaining its applications, benefits, and limitations.
Understanding Pointwise Mutual Information
Pointwise Mutual Information (PMI) is a statistical measure that quantifies the association between two events. Unlike correlation, which measures linear relationships, PMI assesses the degree to which the occurrence of one event influences the probability of another, regardless of the relationship's linearity. This makes it particularly useful when dealing with non-linear relationships that standard correlation methods might miss.
Mathematically, PMI is defined as:
PMI(X, Y) = log₂[P(X, Y) / (P(X) * P(Y))]
Where:
- P(X, Y) is the joint probability of events X and Y occurring together.
- P(X) is the probability of event X occurring.
- P(Y) is the probability of event Y occurring.
A positive PMI value indicates a positive association between X and Y – the presence of one event increases the likelihood of the other. Conversely, a negative PMI suggests a negative association (one event's presence decreases the likelihood of the other). A PMI of zero implies no association.
PMI vs. Correlation: Key Differences
While both PMI and correlation assess relationships, their approaches differ significantly:
- Linearity: Correlation measures linear relationships, while PMI detects any type of association, linear or non-linear.
- Scale: Correlation is sensitive to the scale of variables, whereas PMI is scale-invariant.
- Data Type: Correlation typically works best with continuous data, while PMI is applicable to both continuous and categorical data.
Applications of Pointwise Mutual Information
The versatility of PMI makes it a valuable asset across numerous fields:
1. Natural Language Processing (NLP):
PMI is extensively used in NLP for tasks such as:
- Word association analysis: Identifying semantically related words.
- Collocation extraction: Finding frequently co-occurring words.
- Topic modeling: Discovering latent themes in text corpora.
2. Bioinformatics:
In bioinformatics, PMI assists in:
- Gene co-expression analysis: Determining genes that are expressed together.
- Protein-protein interaction prediction: Identifying proteins that likely interact.
3. Recommender Systems:
PMI helps in building better recommender systems by:
- Identifying item associations: Suggesting products frequently purchased together.
- Personalizing recommendations: Tailoring suggestions based on user preferences.
Advantages of Using Pointwise Mutual Information
- Detects non-linear relationships: Uncovers associations missed by traditional methods.
- Scale-invariant: Provides consistent results regardless of data scaling.
- Applicable to various data types: Works effectively with both continuous and categorical data.
- Provides a clear measure of association strength: Quantifies the strength of the relationship between events.
Limitations of Pointwise Mutual Information
- Sensitivity to low probabilities: PMI can be unstable when dealing with events having very low probabilities. Smoothing techniques are often employed to mitigate this issue.
- Computational cost: Calculating PMI for large datasets can be computationally intensive. Efficient algorithms and approximations are necessary for scalability.
- Interpretation challenges: While PMI provides a quantitative measure, interpreting the magnitude of the association might require domain expertise.
Conclusion: Harnessing the Power of PMI
Pointwise Mutual Information offers a powerful approach to data analysis, providing valuable insights beyond what traditional methods can offer. Its ability to detect both linear and non-linear relationships, combined with its applicability to diverse data types, makes it an indispensable tool for researchers and analysts seeking a deeper understanding of their data. By understanding its strengths and limitations, you can effectively leverage PMI to unlock hidden patterns and make more informed decisions. Remember to consider the context of your data and choose the appropriate methods for analyzing relationships between variables. While PMI provides a robust approach, it often works best in conjunction with other analytical techniques for a comprehensive understanding of your data.
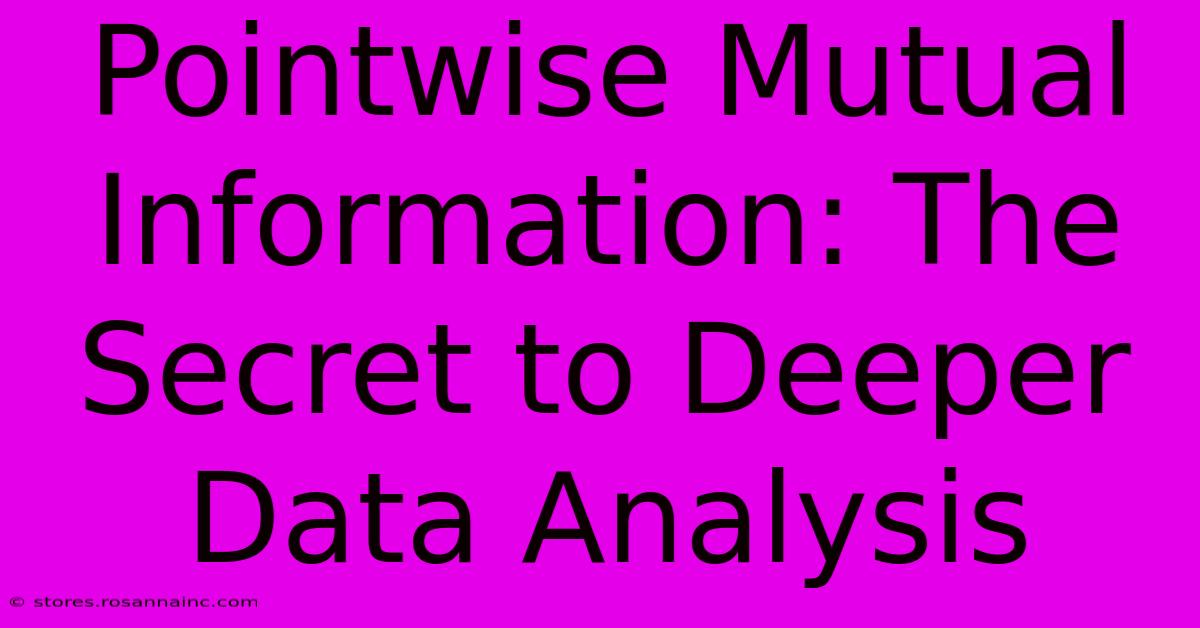
Thank you for visiting our website wich cover about Pointwise Mutual Information: The Secret To Deeper Data Analysis. We hope the information provided has been useful to you. Feel free to contact us if you have any questions or need further assistance. See you next time and dont miss to bookmark.
Featured Posts
-
Why Muhalabieh Matters Understanding Its Cultural Significance
Feb 09, 2025
-
The Ultimate Guide To 3rd Grade Age Requirements
Feb 09, 2025
-
Area Code 770 Discover Its Hidden Gems
Feb 09, 2025
-
Amnh Sadat Dhbyh Pwr Hqyqt Psht Prdh Chyst
Feb 09, 2025
-
Is 604 Your Area Code Heres What You Need To Know
Feb 09, 2025