Stop Guessing, Start Knowing: Pointwise Mutual Information Explained
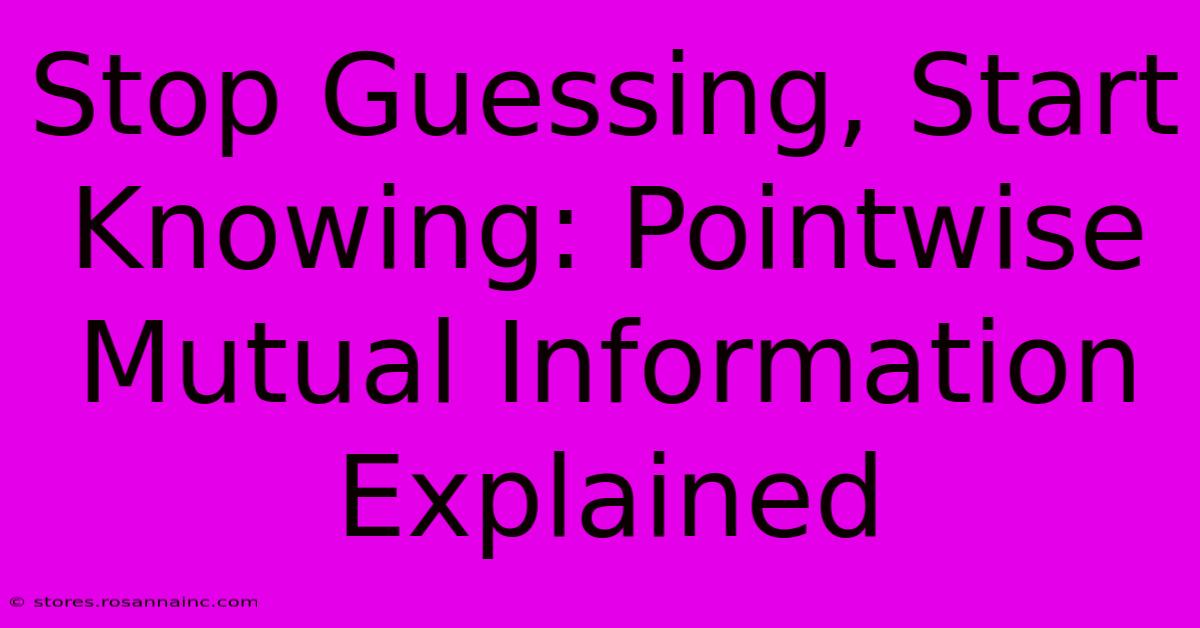
Table of Contents
Stop Guessing, Start Knowing: Pointwise Mutual Information Explained
In the world of data analysis and machine learning, understanding relationships between variables is crucial. Often, we rely on intuition or correlation to assess these relationships, but these methods can be misleading. A more robust and informative measure is Pointwise Mutual Information (PMI). This article will demystify PMI, explaining its calculation, interpretation, and applications.
What is Pointwise Mutual Information (PMI)?
PMI quantifies the amount of information obtained about one random variable, given another. In simpler terms, it measures how much knowing one event tells us about another. Unlike correlation, which measures linear relationships, PMI captures both linear and non-linear associations. A high PMI indicates a strong association; a low PMI suggests a weak or no association; and a negative PMI implies an inverse relationship.
Understanding the Basics: Probability and Information Theory
Before diving into PMI's formula, let's briefly touch upon the fundamental concepts:
- Probability (P): The likelihood of an event occurring. For example, P(A) represents the probability of event A.
- Joint Probability (P(A,B)): The probability of both events A and B occurring simultaneously.
- Conditional Probability (P(A|B)): The probability of event A occurring, given that event B has already occurred.
- Information Content: The amount of surprise or uncertainty associated with an event. A less likely event carries more information. It's calculated as -log₂(P(A)).
Calculating Pointwise Mutual Information
The formula for PMI between two events A and B is:
PMI(A, B) = log₂[P(A, B) / (P(A) * P(B))]
Let's break it down:
- P(A, B): The joint probability of A and B.
- P(A) * P(B): The product of the individual probabilities of A and B. This represents the expected joint probability if A and B were independent.
The ratio inside the logarithm compares the observed joint probability to the expected joint probability under independence. If the events are independent, the ratio will be 1, and the PMI will be 0 (log₂(1) = 0). If the events are positively associated, the ratio will be greater than 1, resulting in a positive PMI. Conversely, a negative association leads to a ratio less than 1 and a negative PMI.
Interpreting PMI Values
- PMI = 0: Events A and B are independent. Knowing one tells us nothing about the other.
- PMI > 0: Events A and B are positively associated. Knowing one increases the probability of the other. The higher the PMI, the stronger the association.
- PMI < 0: Events A and B are negatively associated (inverse relationship). Knowing one decreases the probability of the other.
Applications of Pointwise Mutual Information
PMI finds wide applications in various fields:
- Natural Language Processing (NLP): Identifying word collocations and relationships between words in text corpora. This is crucial for tasks like machine translation, text summarization, and information retrieval.
- Recommender Systems: Determining the association between items or users to provide personalized recommendations.
- Bioinformatics: Analyzing gene expression data and identifying relationships between genes.
- Image Processing: Detecting patterns and relationships in image data.
Limitations of PMI
While powerful, PMI has some limitations:
- Sensitivity to low probabilities: PMI can be unstable when dealing with events with very low probabilities. Small fluctuations in counts can lead to large changes in PMI. Techniques like smoothing can mitigate this issue.
- Doesn't capture higher-order relationships: PMI primarily focuses on pairwise relationships. It doesn't directly capture complex interactions involving more than two variables.
- Difficulty in interpretation with many values: While a single PMI value is easy to interpret, comparing many PMI values across a large dataset can be challenging.
Conclusion
Pointwise Mutual Information offers a powerful and nuanced way to analyze relationships between variables. By understanding its calculation and interpretation, you can move beyond simple correlations and gain deeper insights from your data. While it has some limitations, its versatility and ability to uncover both linear and non-linear relationships make PMI an invaluable tool in diverse fields. Stop guessing; start knowing with PMI!
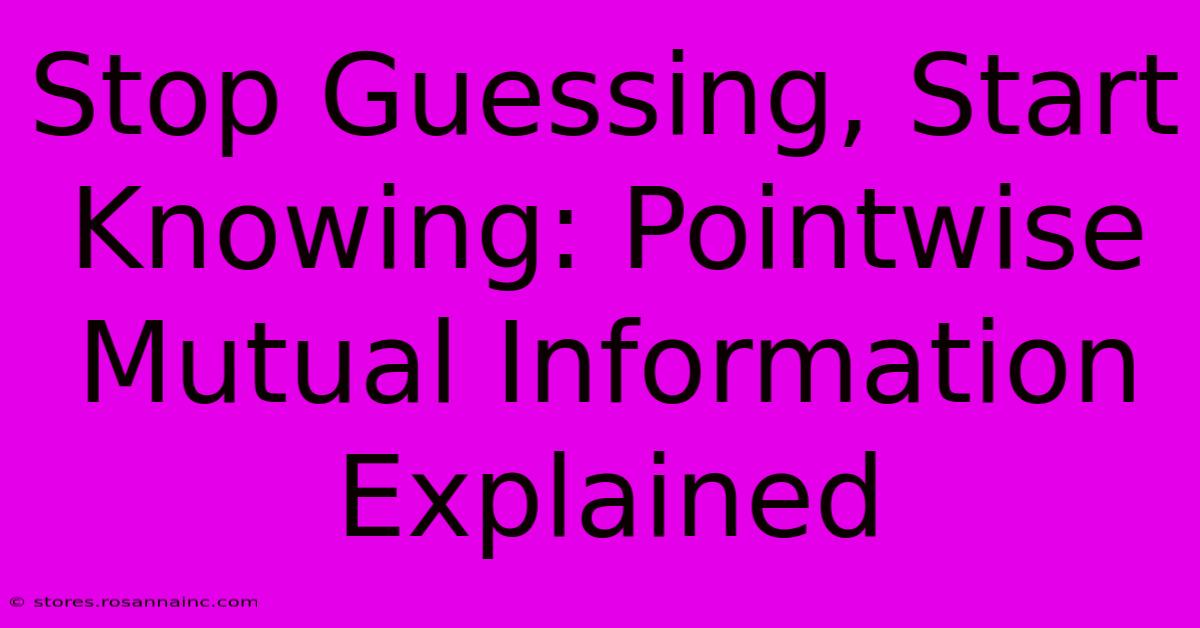
Thank you for visiting our website wich cover about Stop Guessing, Start Knowing: Pointwise Mutual Information Explained. We hope the information provided has been useful to you. Feel free to contact us if you have any questions or need further assistance. See you next time and dont miss to bookmark.
Featured Posts
-
The Shocking Truth Hidden In Albert Fishs X Rays
Feb 10, 2025
-
Super Bowl Anthem The Singer
Feb 10, 2025
-
Jake Pauls Body Transformation From Vine Star To Boxer
Feb 10, 2025
-
Trumps Height Revealed Is It Taller Than You Think
Feb 10, 2025
-
Can Chase Daniel Revive The Saints Offense
Feb 10, 2025