The Secret Weapon For Robust Statistical Analysis: GMM
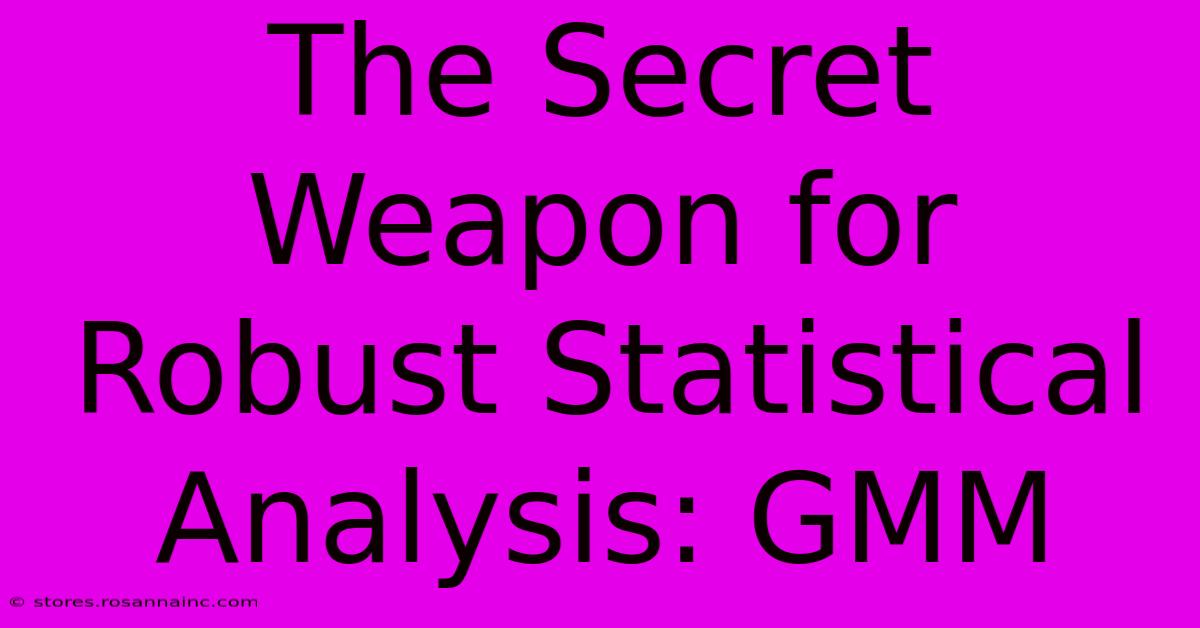
Table of Contents
The Secret Weapon for Robust Statistical Analysis: Generalized Method of Moments (GMM)
For researchers grappling with complex econometric models and limited data, the Generalized Method of Moments (GMM) stands out as a powerful and versatile estimation technique. Often overlooked in favor of more familiar methods like OLS, GMM offers a robust solution, particularly when dealing with issues like endogeneity, autocorrelation, and heteroskedasticity. This article delves into the core principles of GMM, its advantages, limitations, and practical applications.
Understanding the Essence of GMM
GMM is a powerful estimation technique that leverages moment conditions to estimate model parameters. Unlike OLS, which relies on strong assumptions about the error term, GMM requires only that certain moment conditions are satisfied. These conditions are based on the theoretical properties of the model and the data. Essentially, GMM finds parameter estimates that minimize the difference between the sample moments and their theoretical counterparts.
Key Advantages of GMM
-
Robustness: GMM's strength lies in its ability to handle various forms of misspecification. Unlike OLS, it doesn't assume normally distributed errors or homoskedasticity. This makes it highly robust to violations of classical linear regression assumptions.
-
Flexibility: Applicable to a wide range of models, including nonlinear models and models with endogenous variables. This flexibility makes GMM a versatile tool for researchers across numerous disciplines.
-
Efficiency: While robust, GMM is also asymptotically efficient under correct specification. This means that as the sample size grows, the GMM estimates converge to the true parameter values at the fastest possible rate.
-
Handling Endogeneity: One of GMM's most significant advantages is its ability to address endogeneity, a common issue where the explanatory variables are correlated with the error term. Through the use of instrumental variables, GMM provides consistent estimates even in the presence of endogeneity.
The Mechanics of GMM Estimation
The GMM estimation process involves several key steps:
-
Defining Moment Conditions: This involves formulating equations based on the model's theoretical properties. These equations should equal zero in expectation.
-
Choosing Instruments: If dealing with endogeneity, relevant instruments are required. These are variables that are correlated with the endogenous variables but uncorrelated with the error term.
-
Constructing the GMM Objective Function: This function measures the distance between the sample moments and their theoretical counterparts. The goal is to minimize this distance.
-
Optimizing the Objective Function: This typically involves iterative numerical optimization techniques. The parameter values that minimize the objective function are the GMM estimates.
-
Testing the Model: Once estimated, the model needs to be assessed for validity. This involves testing the validity of the overidentifying restrictions. If the model is correctly specified, these restrictions should not be significantly violated.
Limitations of GMM
While powerful, GMM has limitations:
-
Computational Complexity: GMM estimation can be computationally intensive, particularly with large datasets or complex models.
-
Sensitivity to Instrument Choice: The choice of instruments significantly impacts the results. Poor instrument choices can lead to inefficient or inconsistent estimates.
-
Weak Instruments: Weak instruments, which are only weakly correlated with the endogenous variables, can lead to imprecise and unreliable estimates.
Practical Applications of GMM
GMM finds broad applications in various fields:
-
Econometrics: Analyzing economic relationships, particularly when dealing with endogeneity and panel data.
-
Finance: Modeling asset pricing, estimating risk, and forecasting financial markets.
-
Biostatistics: Analyzing longitudinal data and modeling complex biological processes.
Conclusion: GMM – A Powerful Tool in Your Arsenal
The Generalized Method of Moments is a powerful and versatile statistical technique, providing a robust and efficient approach to parameter estimation. Its ability to handle endogeneity and other violations of classical assumptions makes it invaluable for researchers across diverse disciplines. While it presents some computational complexities and requires careful consideration of instrument choice, mastering GMM significantly enhances the robustness and reliability of statistical analyses. By understanding its strengths and limitations, researchers can effectively leverage GMM to gain deeper insights from their data.
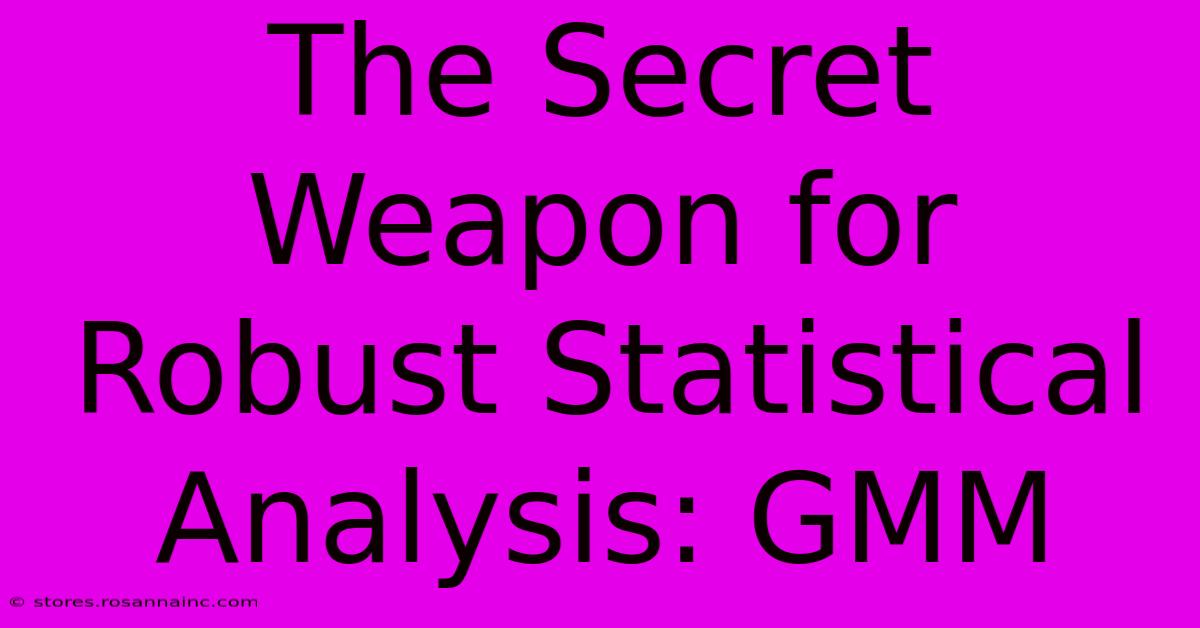
Thank you for visiting our website wich cover about The Secret Weapon For Robust Statistical Analysis: GMM. We hope the information provided has been useful to you. Feel free to contact us if you have any questions or need further assistance. See you next time and dont miss to bookmark.
Featured Posts
-
Unlock Your Next Read House Of The Scorpion
Feb 11, 2025
-
Yeezy Brand Swastika T Shirt Fallout
Feb 11, 2025
-
Unlocking The Mystery What Is A Field Goal In Basketball
Feb 11, 2025
-
Discover Port Moresby Papua New Guineas Hidden Gem
Feb 11, 2025
-
Pff Analyzes Garrett Samuel Trades
Feb 11, 2025