Unlocking Insights With Pointwise Mutual Information
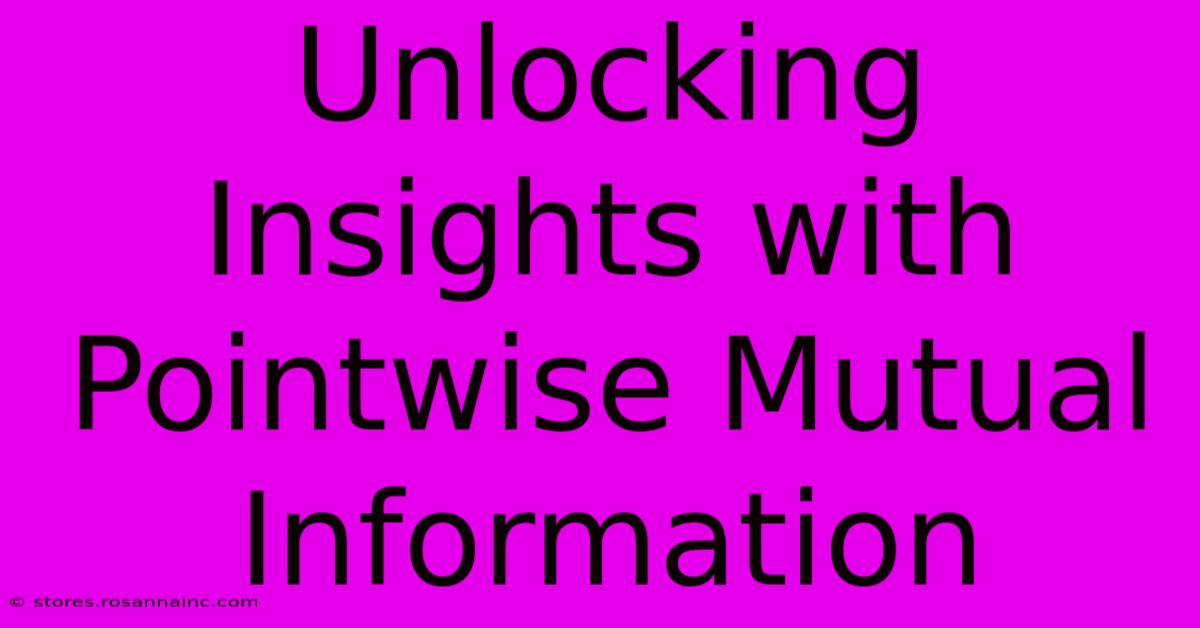
Table of Contents
Unlocking Insights with Pointwise Mutual Information
Pointwise Mutual Information (PMI) is a powerful statistical measure used to quantify the association between two events. It's particularly valuable in fields like natural language processing, information retrieval, and bioinformatics where understanding relationships between words, concepts, or biological entities is crucial. This article will delve into the intricacies of PMI, explaining its calculation, interpretation, and applications.
Understanding Pointwise Mutual Information
PMI measures the amount of information obtained about one random variable when the value of another random variable is known. In simpler terms, it assesses how much knowing one thing tells you about another. A high PMI value indicates a strong association; a low value suggests a weak or no association, while a negative PMI suggests an inverse relationship.
The Formula: Decoding the Calculation
The formula for PMI is deceptively simple yet powerful:
PMI(x, y) = log₂[P(x, y) / (P(x) * P(y))]
Where:
- P(x, y) is the joint probability of events x and y occurring together.
- P(x) is the probability of event x occurring.
- P(y) is the probability of event y occurring.
- log₂ is the logarithm base 2. This is used because it gives the result in bits of information.
Let's break this down:
- P(x, y) / (P(x) * P(y)): This ratio compares the observed joint probability to the expected joint probability if x and y were independent. If x and y are independent, this ratio will be 1, and the PMI will be 0.
- log₂: This function transforms the ratio into a more interpretable scale. A PMI of 1 bit means that knowing x doubles the probability of y (or vice versa). A PMI of 2 bits quadruples the probability, and so on.
Applications of Pointwise Mutual Information
PMI's versatility makes it applicable across numerous domains:
1. Natural Language Processing (NLP):
-
Word association: PMI is frequently used to identify strong relationships between words in text corpora. This information is valuable for tasks like synonym detection, collocation extraction, and building semantic networks. Consider analyzing the PMI between "sun" and "shine" – it would likely be high, indicating a strong association.
-
Topic modeling: PMI can help uncover latent topics within large text datasets by measuring the co-occurrence of words.
2. Information Retrieval:
-
Query expansion: By identifying words strongly associated with search terms, PMI can enhance the effectiveness of information retrieval systems.
-
Relevance ranking: PMI can help rank documents based on their relevance to a given query by analyzing the co-occurrence of query terms and document words.
3. Bioinformatics:
-
Gene co-expression: PMI can be used to identify genes that are likely to be co-regulated based on their expression patterns across different samples.
-
Protein-protein interaction: PMI can aid in the prediction of protein-protein interactions by analyzing the co-occurrence of proteins in various biological pathways or datasets.
Limitations of PMI
While PMI is a valuable tool, it's essential to be aware of its limitations:
-
Sparsity: PMI can be unreliable when dealing with low frequency events. The probabilities involved in the calculation might be poorly estimated, leading to inaccurate results. Techniques like smoothing can help mitigate this issue.
-
Sensitivity to corpus size: PMI values can vary depending on the size and nature of the corpus used to estimate the probabilities.
-
Does not consider contextual information: PMI only considers the co-occurrence of events and doesn't capture the context in which they appear.
Beyond Basic PMI: Addressing Limitations
Researchers have developed variations of PMI to overcome some of these limitations. These include:
-
Positive Pointwise Mutual Information (PPMI): This variant sets negative PMI values to zero, focusing only on positive associations.
-
Normalized Pointwise Mutual Information (NPMI): This normalization addresses the problem of high PMI values for low-probability events.
Conclusion: Harnessing the Power of PMI
Pointwise Mutual Information is a valuable technique for uncovering hidden relationships between events. While it has limitations, understanding its strengths and weaknesses allows for effective application across a wide range of disciplines. By carefully considering the context and addressing potential biases, researchers can harness the power of PMI to unlock valuable insights from data. Remember to choose the appropriate PMI variant and address data sparsity for accurate and reliable results.
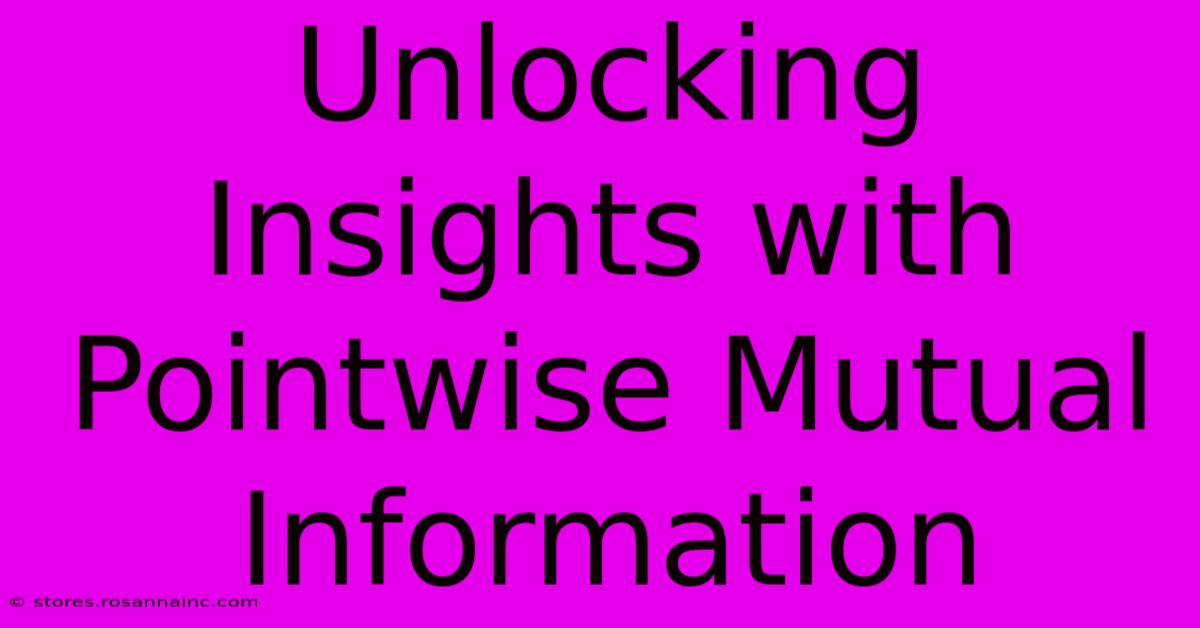
Thank you for visiting our website wich cover about Unlocking Insights With Pointwise Mutual Information. We hope the information provided has been useful to you. Feel free to contact us if you have any questions or need further assistance. See you next time and dont miss to bookmark.
Featured Posts
-
Seisme 7 6 Alerte Tsunami
Feb 09, 2025
-
Will Character Name Return The Gilded Age Season 3 Predictions
Feb 09, 2025
-
Solution De Cotillard Apres Blessure
Feb 09, 2025
-
Escape From The Habsburgs Archduchess Elisabeth Maries Fight For Freedom
Feb 09, 2025
-
Conquer Your Boredom Drag Race Uk Season 6 Is Here
Feb 09, 2025