Unlocking Insights With The Generalized Method Of Moments
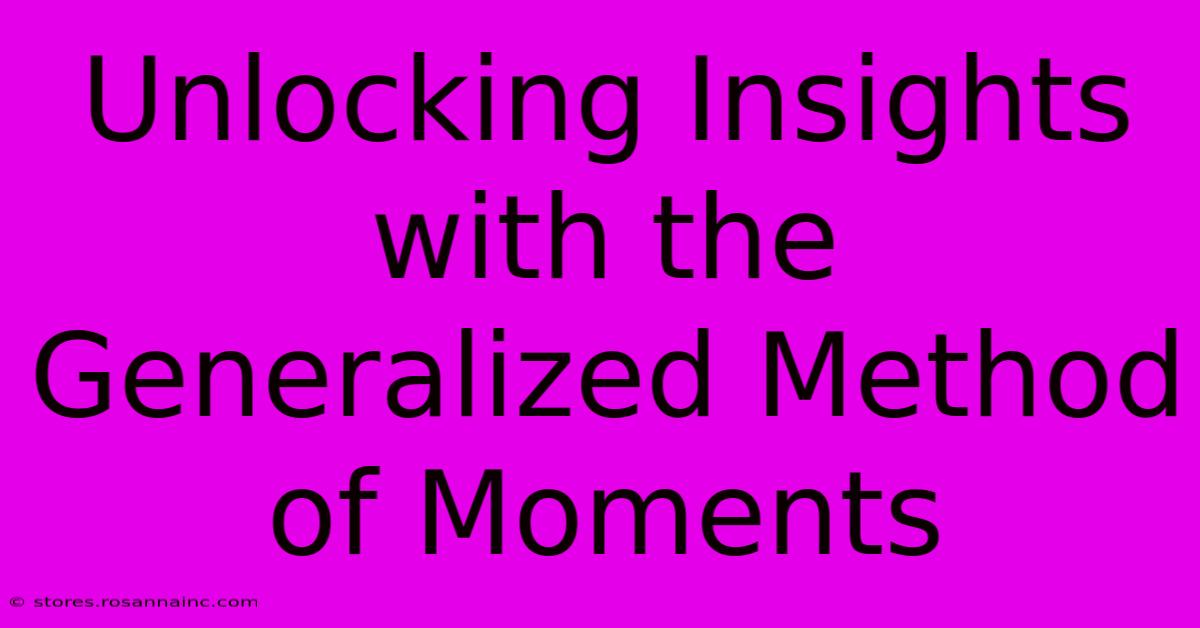
Table of Contents
Unlocking Insights with the Generalized Method of Moments (GMM)
The Generalized Method of Moments (GMM) is a powerful statistical technique used to estimate parameters in econometric models and other statistical settings. It's particularly valuable when dealing with situations where traditional methods like maximum likelihood estimation (MLE) are difficult or impossible to apply. This article will explore the fundamentals of GMM, its advantages, and its applications.
What is the Generalized Method of Moments?
GMM is a flexible estimation method that leverages moment conditions. Moment conditions are equations that specify the expected value of certain functions of the data and the model parameters. These conditions are derived from the underlying economic theory or assumptions about the data generating process. The core idea is to find parameter estimates that make the sample moments as close as possible to the theoretical moments specified in the conditions. This minimization is accomplished using a weighting matrix.
Key Components of GMM:
- Moment Conditions: These are the heart of GMM. They express the relationships between the data and the unknown parameters. Correctly specifying these conditions is crucial for accurate estimation.
- Weighting Matrix: This matrix determines the relative importance given to different moment conditions during the estimation process. The optimal weighting matrix is crucial for efficient estimation. Often, this is a function of the estimated parameters themselves, requiring an iterative procedure.
- Objective Function: GMM minimizes a distance metric between the sample moments and the theoretical moments. The specific metric used depends on the choice of weighting matrix.
Advantages of GMM:
- Flexibility: GMM can handle a wider range of models and data structures than MLE. It doesn't require full specification of the likelihood function, making it applicable in situations with complex data or incomplete information.
- Robustness: GMM is less sensitive to misspecification of the error distribution than MLE. This robustness is a significant advantage when the true distribution of the errors is unknown or difficult to model.
- Efficiency: When the optimal weighting matrix is used, GMM yields asymptotically efficient estimators. This means that the estimates have the smallest possible variance in large samples.
Applications of GMM:
GMM has found wide applications across numerous fields, including:
- Econometrics: Estimating dynamic panel data models, simultaneous equations models, and models with endogenous variables. It's particularly useful in situations where instrumental variables are needed.
- Finance: Estimating asset pricing models, volatility models, and models of market microstructure.
- Biostatistics: Analyzing longitudinal data and survival data.
Limitations of GMM:
Despite its advantages, GMM is not without limitations.
- Over-identification: When the number of moment conditions exceeds the number of parameters, the model is over-identified. This can lead to testing for the validity of the over-identifying restrictions. A significant test statistic may indicate misspecification of the model.
- Computational Complexity: GMM estimation often requires iterative procedures, which can be computationally intensive, especially with large datasets.
- Sensitivity to Initial Values: The choice of initial values for the parameters can affect convergence.
Conclusion:
The Generalized Method of Moments is a versatile and powerful tool for parameter estimation in a wide range of statistical models. Its flexibility, robustness, and efficiency make it a valuable asset in situations where traditional methods are insufficient. Understanding its underlying principles, advantages, limitations, and applications is crucial for researchers across various disciplines. However, careful consideration of model specification and potential challenges, such as over-identification, is vital for achieving reliable results. Further exploration into the specific applications and variations of GMM within your field of interest will enhance your ability to leverage this valuable statistical technique.
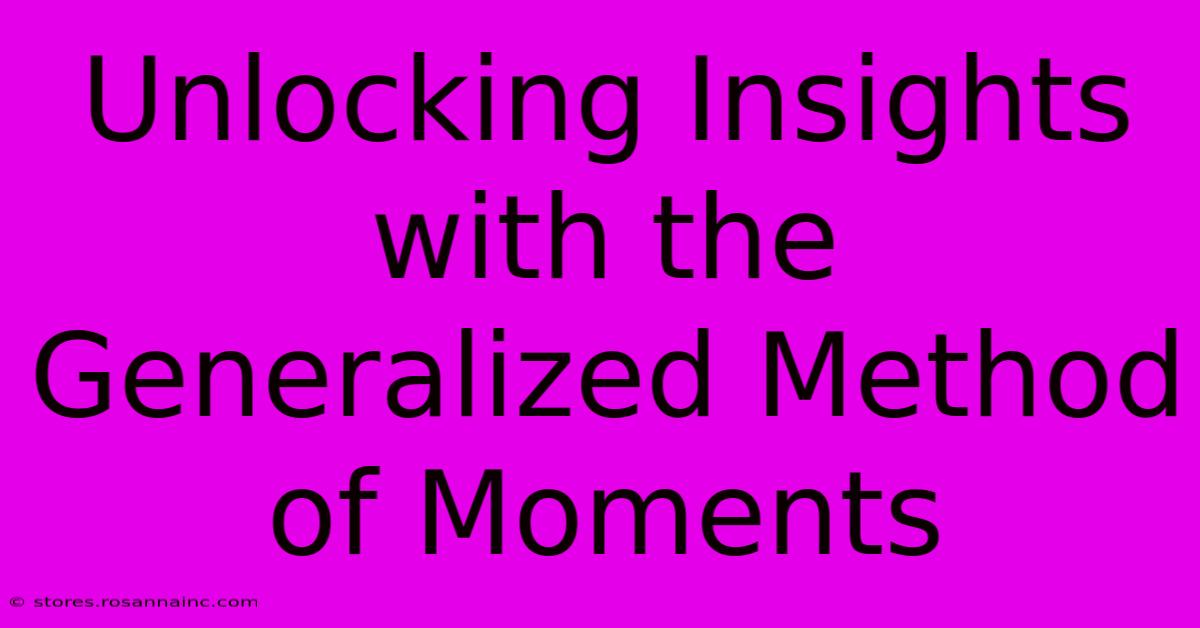
Thank you for visiting our website wich cover about Unlocking Insights With The Generalized Method Of Moments. We hope the information provided has been useful to you. Feel free to contact us if you have any questions or need further assistance. See you next time and dont miss to bookmark.
Featured Posts
-
The Billion Dollar Question Whos At The Helm Of The Ufc
Feb 09, 2025
-
Laugh Out Loud Dwights Shining Armor Adventure
Feb 09, 2025
-
19 Second Ko Salkilld Ufc 312
Feb 09, 2025
-
Halfway House A Fresh Start After Difficult Times
Feb 09, 2025
-
Is Bruno Mars A Billionaire Net Worth Finally Uncovered
Feb 09, 2025